Enhance your data capabilities
It is difficult to hire technologists, and hiring practitioners in the continually evolving science of data and analytics even more so. Let us examine whether you need data scientists, or, like how computer science has given way to software development, has data science paved the way for hands-on practitioners to take over?
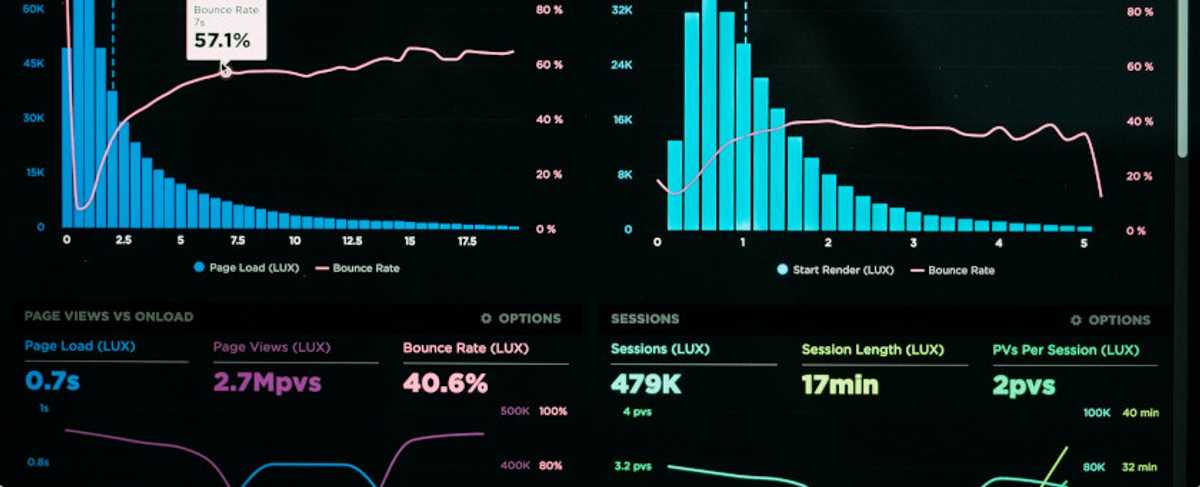
Last week at the first Virtual CxO Breakfast Club I had an opportunity to opine about the evolution of computer science jobs, and how data science has rapidly followed suit. Namely, very few organizations hire computer scientists, even though the prevalent degree is “computer science” – organizations hire software engineers, developers, infrastructure managers, etc. In my opinion we should be thinking about data science in this fashion as well and update our thinking on the type of data practitioners we are needing.
The state of computer science is such that building digital products and experiences requires artists and engineers to build upon the solid foundations that computer scientists have provided, and few organizations are working on (or even need to contemplate) updating that foundation. For most current needs, data science has reached the level of maturity where deep exploration and trial and error are no longer necessary. Analysts and engineers can utilize existing tools and services to provide insight and intelligence to the business, even including advanced predictive and machine learning features.
Over the past two decades data scientists have used sophisticated tools such as SAS or R to create clustering models, regressions, and other advanced sophisticated statistics. They were able to leverage big data to enhance model precision and scale it to every customer, citizen, or potential customer in the world. They created these models to generate “lift” predictions, i.e., the additional revenue possible by undertaking certain product actions. In early web-based incarnations we saw fruits such as the “recommendations bar” (aka cross-selling) and targeted coupons and circulars. Today you are just as likely to be using statistical techniques to understand the likely outcome of product launches or performing sentiment analyses on social media posts.
But look at the plethora of tools available today! If you put data into the cloud you have a wealth of analytical power at your fingertips, and your engineers can compose data capabilities for you that would astound the data scientists of a few years ago. Depending on your cloud provider you might want to take an engineering-centric approach or a more graphical approach. Maybe you are wanting nice dashboards or reporting? Keeping your data at home? The world of data is your analytical oyster.
Before you get out the pitchforks, I am not saying data scientists are not needed. Many tools require extensive understanding of statistics, programming, and data engineering, and data scientists can provide that sweet spot. Additionally, industries only break new ground through the significant efforts of academics and scientists and their rigorous experimentation. However, I have seen several competing factors that make hiring and keeping data scientists more and more of a challenge:
- Data scientists switch jobs frequently because of expectation mismatches
- Data engineers are the appropriate experts for data management and movement
- Business experts are in the appropriate spot to utilize data insights, and most industries have by now recognized the need for additional training in data and statistics
Putting this all together it is obvious to me that instead of hiring data scientists, you will want to focus on hiring data ENGINEERS and ANALYSTS. Your engineers will work with the data that your product team is gathering and build out your data lake/warehouse/etc. and create efficient streams to turn your transactional data into intelligence data. Your analysts will take this data and utilize available tools to create both point-in-time information as well as predictive insights. They will not need to build sophisticated machine learning techniques and experiment endlessly to eke additional marginal lift and boost but will instead leverage tools created by some of the sharpest minds in the business. Just like how software engineers and business analysts can work with subject matter experts to create compelling digital products, the data industry is now mature enough to allow engineers and analysts to work with the business to create compelling, actionable insights.